The SIGDial venue provides a regular forum for the presentation of cutting edge research in discourse and dialogue to both academic and industry researchers. The conference is sponsored by the SIGDial organization, which serves as the Special Interest Group in discourse and dialogue for both ACL and ISCA. Continuing a series of successful previous meetings, […]
SIGdial is the Special Interest Group on Discourse and Dialogue.
The SIGDIAL venue provides a regular forum for the presentation of cutting edge research in discourse and dialogue to both academic and industry researchers. The proceedings are indexed by Web of Science and hosted on the ACL Anthology.
The 26th Annual Meeting will be held in Avignon, France, from August 25 to August 27, 2025.
Latest News
As you all know, every two years we hold elections for our Executive Committee and Advisory Board. We are looking to fill the following positions: All members serve for 2 years and the executive committee meet bi-weekly. The purpose of the executive committee is to oversee the SIGDial conference, finances, and liaise with other organizations. […]
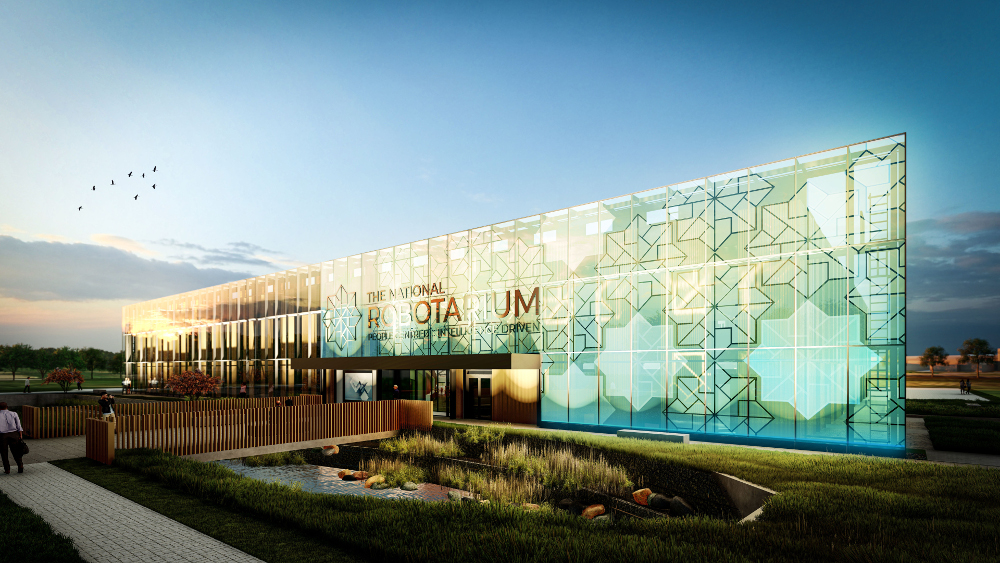
View the CFP on the SIGDial Website. Call for Papers The 23rd Annual Meeting of the Special Interest Group on Discourse and Dialogue (SIGDIAL 2022) will be held as a hybrid conference at Heriot-Watt University in Edinburgh and online between September 7-9, 2022. The SIGDIAL venue provides a regular forum for the presentation of cutting edge research […]