The results are in!
SIGdial is the Special Interest Group on Discourse and Dialogue.
The SIGDIAL venue provides a regular forum for the presentation of cutting edge research in discourse and dialogue to both academic and industry researchers. The proceedings are indexed by Web of Science and hosted on the ACL Anthology.
The 25th Annual Meeting will be held in Kyoto, Japan, from September 18 to September 20, 2024.
Latest News
As you all know, every two years we hold elections for our Executive Committee and Advisory Board. We are looking to fill the following positions: All members serve for 2 years and the executive committee meet bi-weekly. The purpose of the executive committee is to oversee the SIGDial conference, finances, and liaise with other organizations. […]
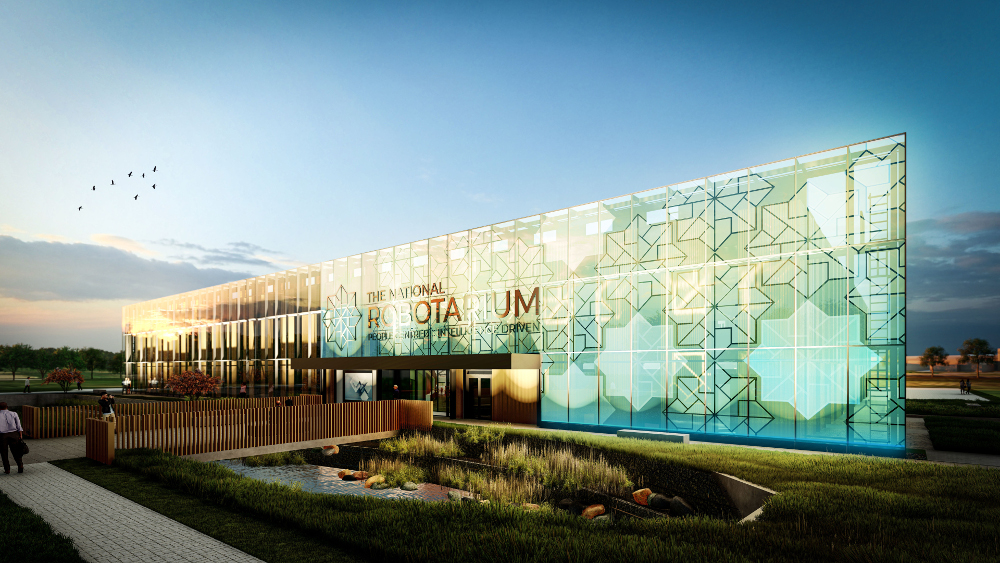
View the CFP on the SIGDial Website. Call for Papers The 23rd Annual Meeting of the Special Interest Group on Discourse and Dialogue (SIGDIAL 2022) will be held as a hybrid conference at Heriot-Watt University in Edinburgh and online between September 7-9, 2022. The SIGDIAL venue provides a regular forum for the presentation of cutting edge research […]
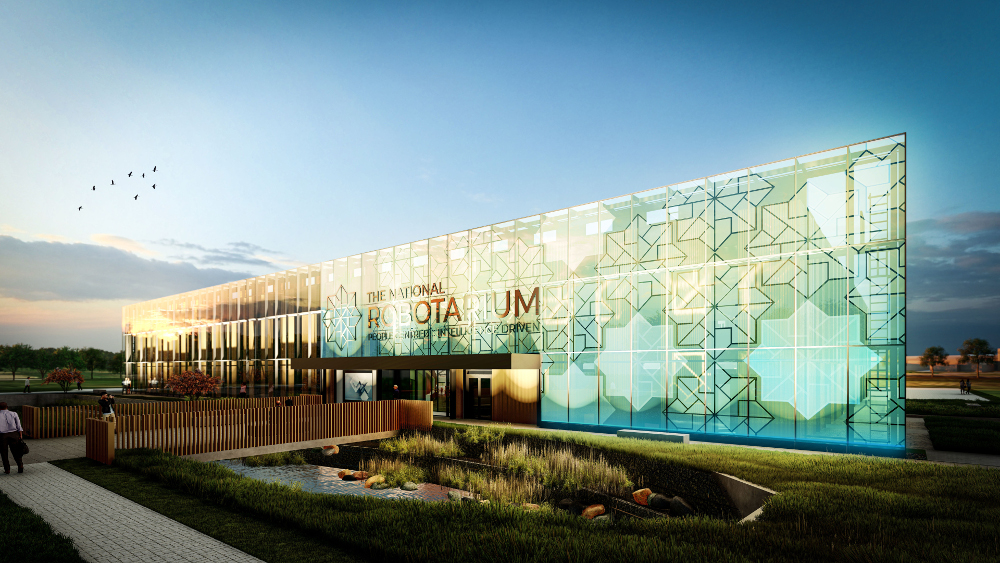
We welcome special session proposals on any topic of interest to the discourse and dialogue communities. Topics of interest include, but are not limited to Role of Discourse in NLP Applications, Explainable AI, Evaluation, Annotation, End‐to‐end systems, Vision and Language, and Human-Robot Interaction.